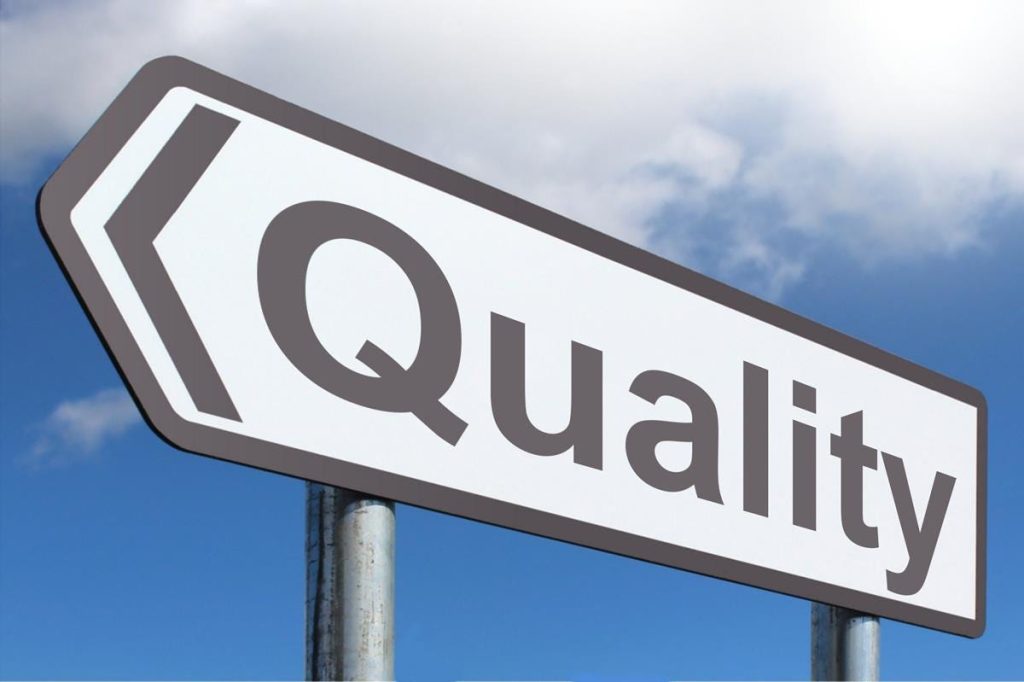
This fairly practical article emphasizes the significance of quality metrics in organizations, offering a methodology for effectively selecting, tracking, and reporting these metrics. The article outlines that quality metrics should be monitored to benefit the enterprise. Good metrics are described as providing an unbiased performance assessment and maintaining a balance between quality, cost, and delivery. The article warns against metrics driving undesirable behaviors, citing the Wells Fargo fake accounts scandal as an example.
The article discusses common errors in metric utilization, such as creating metrics just for the sake of it, having too many metrics leading to inaction, lack of follow-up, and metrics that result in emotional manipulation. The importance of designing and using metrics effectively is stressed to avoid them becoming counterproductive. The selection of data quality metrics should focus on time-series tracking and align with business needs and customer expectations. Where possible, metrics should be updated automatically (e.g., daily) and reported from a process output perspective rather than focusing on individual or departmental goals.
The article introduces predictive performance metric reporting through a 30,000-foot-level reporting approach. This method helps identify issues in meeting monthly targets and differentiates between common-cause and special-cause variability. To determine which metrics to report, the article suggests asking what is essential to customers and focusing on high-level metrics for process improvement efforts. Examples include product return rates, on-time delivery, shipment errors, lead times, internal rework rates, customer satisfaction, warranty claims, product specifications, and non-conformance costs.
Finally, the article explains that a 30,000-foot-level quality report is not just a traditional control chart but takes various formats depending on the data type. This high-level performance reporting includes predictions about whether the process output is predictable and stages the process when a demonstrated improvement occurs. The article highlights the need for transparency and accuracy in reporting systems, discouraging data manipulation, linking metrics to the functional processes that created them, and assigning ownership for each reported metric.
Leave a Reply
You must be logged in to post a comment.