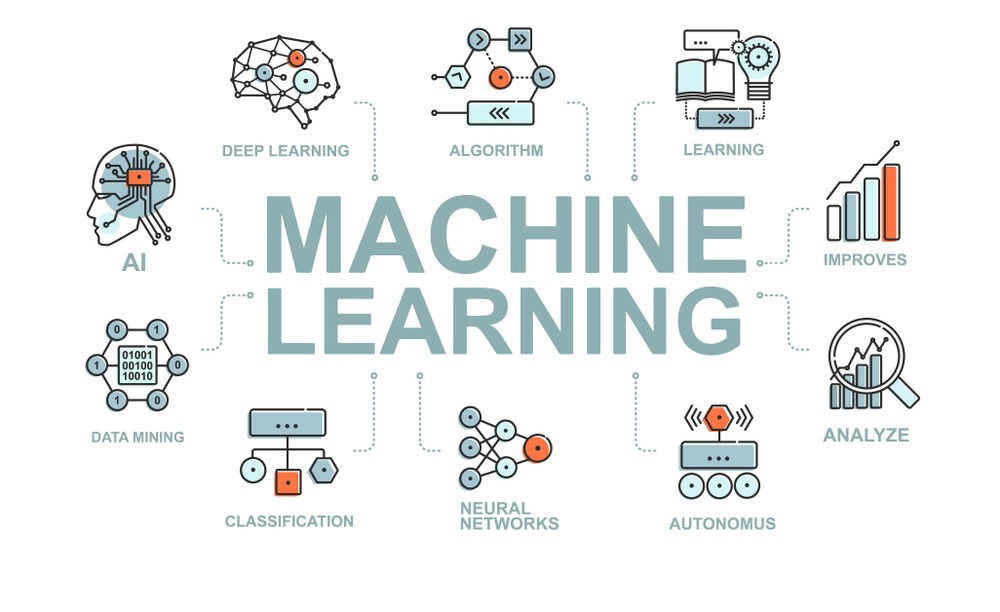
- Random Forest (RF) and Long Short-Term Memory (LSTM) are the most used machine learning models for analyzing IoT data in manufacturing.
- Most studies focus on maintenance data, with few addressing production data.
- The concept of machine learning interpretability is under-explored in the context of manufacturing.
The integration of Internet-of-Things (IoT) data with machine learning (ML) and deep learning (DL) models has the potential to transform manufacturing operations by providing real-time insights and predictions. Industry 4.0, characterized by advanced technologies like IoT and AI, aims to enhance manufacturing efficiency and operational excellence. However, despite the abundance of studies on IoT applications in manufacturing, there is a noticeable gap in the literature concerning the interpretability of these ML models.
A systematic literature review identified that Random Forest (RF) and Long Short-Term Memory (LSTM) models are predominantly used in manufacturing settings. Most of the research has concentrated on maintenance operations, specifically predictive maintenance, leaving other areas, such as production, under-researched. The interpretability of these models, which is crucial for understanding the causal relationships between inputs and outputs, remains largely unexplored. This lack of interpretability can hinder the adoption of ML models in real-world industrial environments.
Interpretability in ML models is essential for gaining trust and enabling informed decision-making in manufacturing operations. While many ML and DL models, especially those used for anomaly detection, are effective, they often function as black boxes, providing little insight into how decisions are made. This study emphasizes the importance of developing interpretable models to explain their predictions, thus supporting continuous improvement strategies and operational excellence.
Future research should focus on incorporating interpretability into ML models used in manufacturing. This involves using intrinsically interpretable models and post-hoc techniques to make complex models more understandable. Enhancing model interpretability will improve transparency and decision-making and facilitate the implementation of continuous improvement initiatives. Additionally, integrating domain knowledge and exploring the trade-off between model accuracy and interpretability can further advance the application of AI in manufacturing, making it a powerful tool for operational excellence.
Leave a Reply
You must be logged in to post a comment.