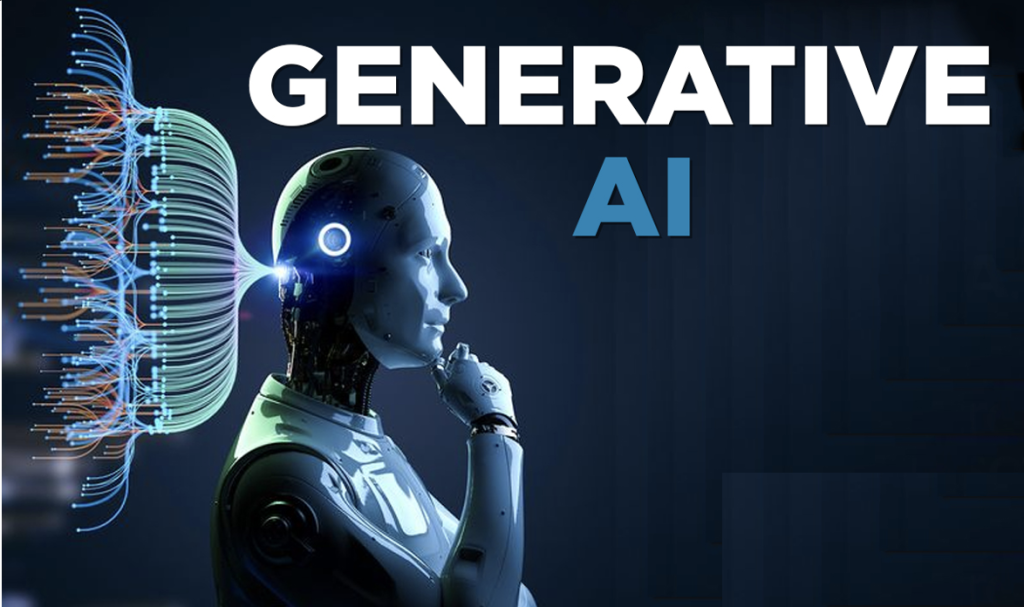
- The concept of non-human identity (NHI) governance is intended to protect data privacy and integrity in applications built on the Retrieval-Augmented Generation (RAG) architecture, which combines large language models (LLMs) with specific data to enhance interactions.
- The intersection of RAG and NHIs, particularly improper management of NHIs, such as unrotated access keys and stale service principals, can lead to data breaches and privacy issues.
- Proper lifecycle management, monitoring, and adherence to best practices are essential to protecting AI training data and ensuring the accuracy and integrity of responses from AI-enabled applications.
Unique risks and security needs are associated with the rapid innovation in generative AI technologies. As businesses seek value from AI-driven applications, ensuring their safe usage and implementation is crucial. The concept of non-human identity (NHI) governance protects data privacy and integrity in applications built on the Retrieval-Augmented Generation (RAG) architecture, which combines large language models (LLMs) with specific data to enhance interactions. Even those who are not involved direclty in AI-enabled models need to understand the risks posed by poor administration of the security needed to support safe practices.
RAG architecture leverages the conversational power of LLMs, like OpenAI’s GPT, with localized data to create robust applications for customer interactions and employee productivity. This architecture relies heavily on NHIs, such as service accounts, tokens, and API keys, facilitating machine-to-machine communication. However, the rapid expansion and often poor governance of NHIs pose significant security risks, especially as they become the primary access control in cloud environments.
The intersection of RAG and NHIs, particularly improper management of NHIs, such as unrotated access keys and stale service principals, can lead to data breaches and privacy issues. For instance, the misuse of storage accounts in cloud environments like Azure can expose sensitive data and increase the risk of data leakage or unauthorized data modifications. This is exemplified by incidents like the Microsoft AI team’s data exposure due to mismanaged SAS tokens.
Robust NHI management is important to mitigate these risks. Proper lifecycle management, monitoring, and adherence to best practices are essential to protecting AI training data and ensuring the accuracy and integrity of responses from AI-enabled applications. Certain software can provide visibility and lifecycle management for NHIs, offering insights into posture issues and automating remediation to safeguard high-value projects and maintain data security.
Leave a Reply
You must be logged in to post a comment.