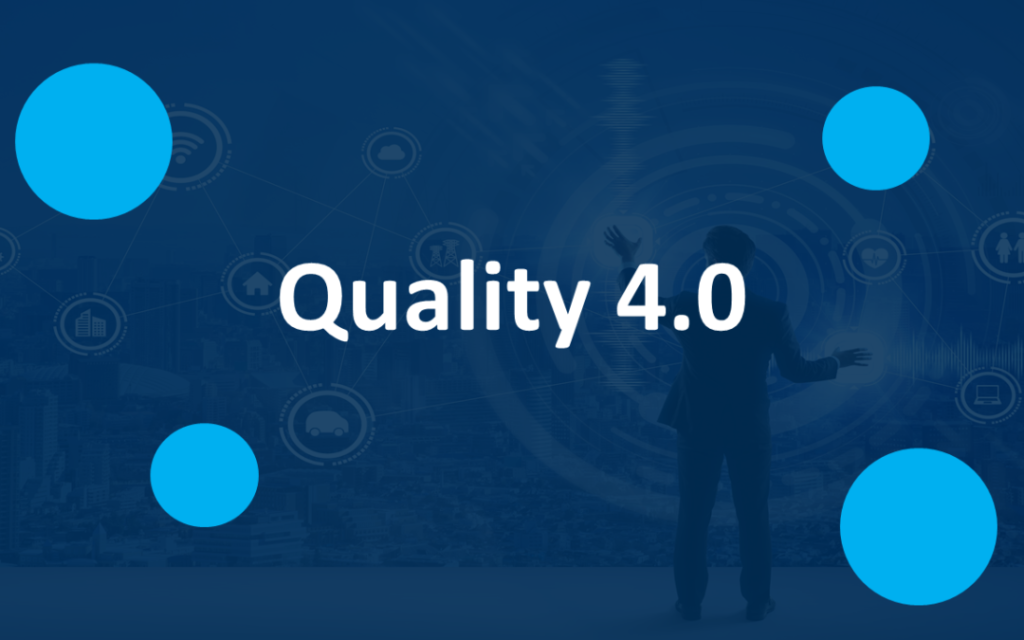
- In this study, XGBoost outperformed other machine learning models in predicting customer complaints, achieving an F1-Score of 72.4% and a Matthews correlation coefficient of 75%.
- The machine learning framework improves the lot-release process by addressing data imbalances and allowing for efficient, flexible adjustments without retraining models.
- Integrating this framework with Quality 4.0 principles optimizes industrial processes, reduces costs, and enhances customer satisfaction through data-driven decision-making.
In this study, a machine learning (ML) framework was analyzed for its ability to predict customer complaints in the lot-release process of an automotive company, enhancing the adoption of Quality 4.0 principles. The framework integrates four ML models, including XGBoost, LightGBM, CatBoost, and Random Forest, to analyze production and repair data. Using cost-sensitive learning and threshold-moving techniques addressed data imbalance, with XGBoost emerging as the most effective model, achieving an F1-Score of 72.4% and a Matthews correlation coefficient of 75%. This approach improves lot-release decisions, reduces customer complaints, and enhances cost efficiency.
The methodology followed the Cross-Industry Standard Process for Data Mining (CRISP-DM), ensuring a structured data collection, preparation, and model evaluation approach. The framework was tested on real-world data, showing promising results in predicting customer complaints and improving the lot-release process. By allowing companies to adjust predictive models through threshold modification without retraining, the framework provides flexibility and efficiency in managing production quality.
The study results highlight the potential of integrating ML with Quality 4.0 strategies to optimize industrial processes, reduce costs, and improve customer satisfaction. The framework’s ability to predict complaints and adjust to specific business needs underscores the importance of data-driven decision-making in modern quality management. Future research should explore larger datasets, alternative ML algorithms, and the economic implications of this approach to validate further and enhance its effectiveness.
Leave a Reply
You must be logged in to post a comment.