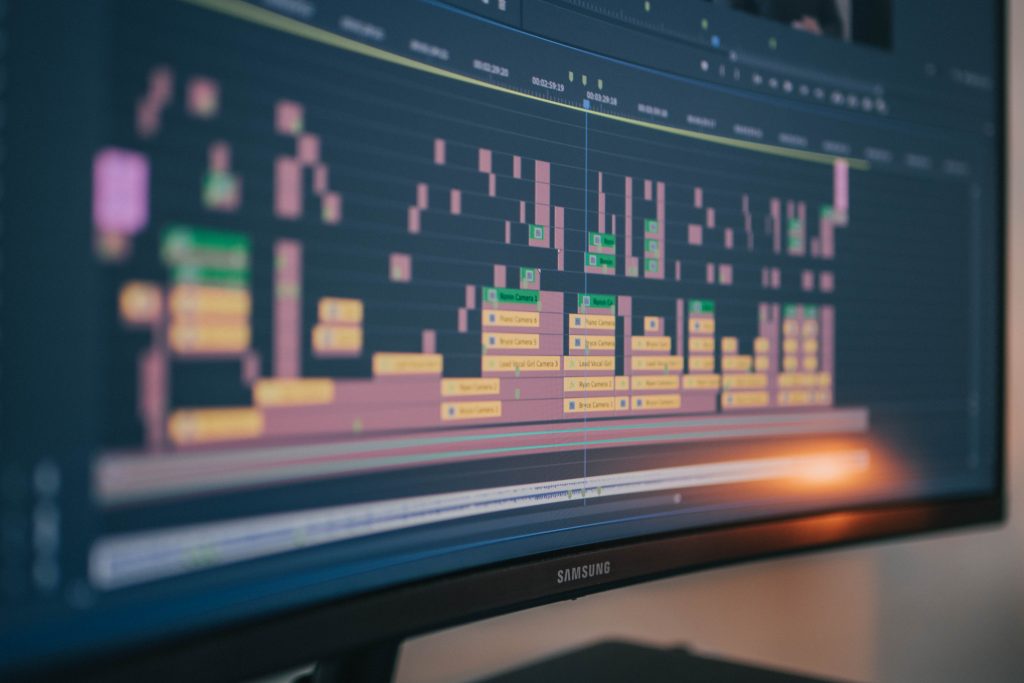
- There are nuances between Data Quality (DQ) and Data Enrichment, each providing separate but their critical roles in business insights and risk management.
- The distinction between DQ and Data Enrichment is that enrichment alone cannot solve all DQ problems. For true DQ improvement, businesses must understand how both concepts complement each other and how enrichment can serve as a DQ tactic.
- When executed properly, Data Enrichment can significantly enhance DQ by providing more detailed insights into data sets.
Manufacturers are collecting information from an ever-broadening network of sources. Whether it’s time-series data from traditional physical sensors, real-time video streams, or unstructured and manually entered reports, data are at the core of day-to-day operational decision making. What are the nuances of Data Quality (DQ) and Data Enrichment? Highlighting their critical roles in business insights and risk management emphasizes that while DQ pertains to the confidence in data’s utility, Data Enrichment involves enhancing existing data sets to improve DQ and derive deeper insights. There is a pressing issue of DQ affecting a significant portion of revenue and the average time to resolve DQ incidents. In response, over half of the organizations plan to implement AI and automation solutions, including data enrichment, to tackle these challenges.
There is a distinction between DQ and Data Enrichment, stressing that enrichment alone cannot solve all DQ problems. For true DQ improvement, businesses must understand how both concepts complement each other and how enrichment can serve as a DQ tactic. The importance of a consistent understanding of DQ across an enterprise is underscored, noting that a unified DQ definition is essential for effectively utilizing data enrichment as a strategy.
So when executed properly, Data Enrichment can significantly enhance DQ by providing more detailed insights into data sets. However, it also presents a cautionary tale illustrating that data enrichment can lead to more complex issues without a robust DQ program in place, making it costlier and more time-consuming to resolve discrepancies. To mitigate such risks, implementing a strong DQ program, serviced by accountable Data Governance, is recommended before pursuing data enrichment initiatives. This approach ensures data integrity and compliance with regulations, paving the way for data enrichment to improve DQ and support business goals meaningfully.
Leave a Reply
You must be logged in to post a comment.