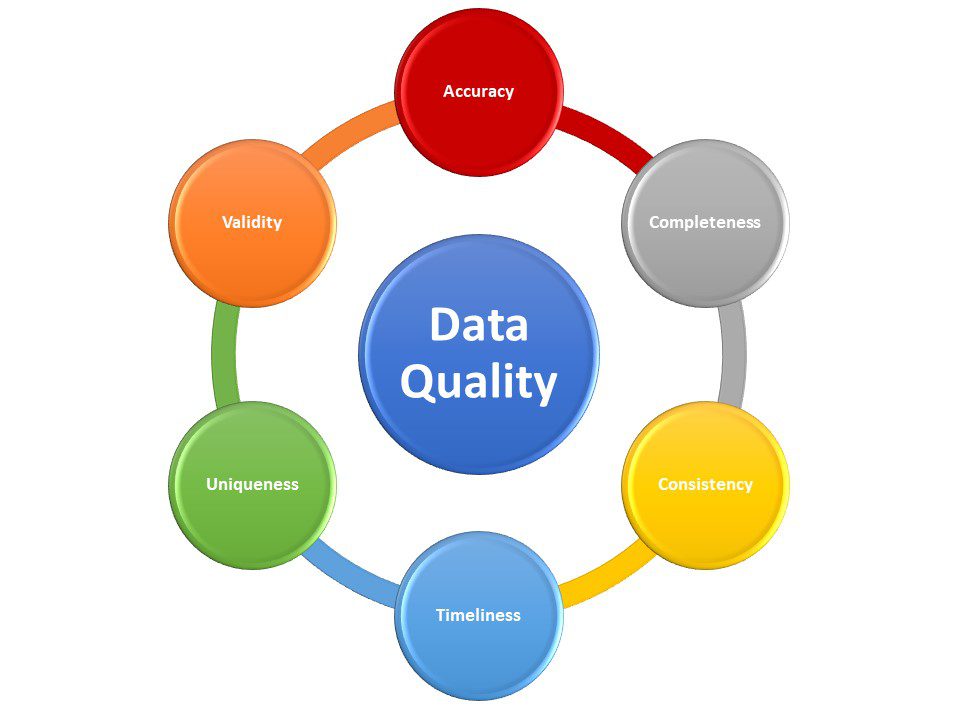
- Data quality management (DQM) ensures data accuracy, integrity, and reliability, allowing marketing teams to focus on strategic decision-making.
- DQM differs from data governance, which focuses on setting policies and compliance standards that support quality data use.
- Implementing effective DQM programs requires setting clear objectives, standardizing procedures, using tools, and fostering a culture of data quality.
Data Quality Management (DQM) is crucial in supporting strategic decision-making for marketing teams by ensuring that the data they rely on is accurate, consistent, and up-to-date. Through practices like data cleansing, validation, and integration, DQM helps teams focus on analyzing and applying insights rather than being bogged down by data inaccuracies. This framework provides a foundation for reliable data that supports essential marketing functions such as customer segmentation and campaign optimization, improving performance and decision-making.
Although closely related, DQM is distinct from data governance. While DQM is tactical, involving data maintenance and quality control, data governance is more strategic, establishing policies, data ownership, and compliance protocols. Data governance sets the standards and framework for DQM, ensuring that data practices align with organizational goals and regulatory requirements. These two functions help maintain data integrity and regulatory compliance, enabling marketing teams to manage data effectively and avoid costly errors.
Effective implementation of DQM requires several vital steps. First, organizations must define clear data quality objectives, specifying what constitutes quality data and aligning these standards with business goals. Standardized procedures for handling data ensure consistency and reduce error risk, especially in environments with high turnover. Advanced DQM tools like Improvado and Cerebro automate data processes and offer real-time monitoring, which improves data reliability and reduces manual data maintenance, allowing marketing teams to focus on strategic insights. Continuous monitoring is vital for identifying and resolving data issues promptly and maintaining the high quality of data over time.
A successful DQM strategy also fosters a data-quality culture throughout the organization. Leadership should advocate for data quality initiatives, and specific teams or individuals can take responsibility for maintaining data standards within their departments. By integrating data quality practices into everyday workflows and utilizing automated tools to flag errors, marketing teams can maintain a high standard of data accuracy. This focus on data quality enables smarter, evidence-based decisions that drive campaign success and ultimately contribute to business growth.
Leave a Reply
You must be logged in to post a comment.