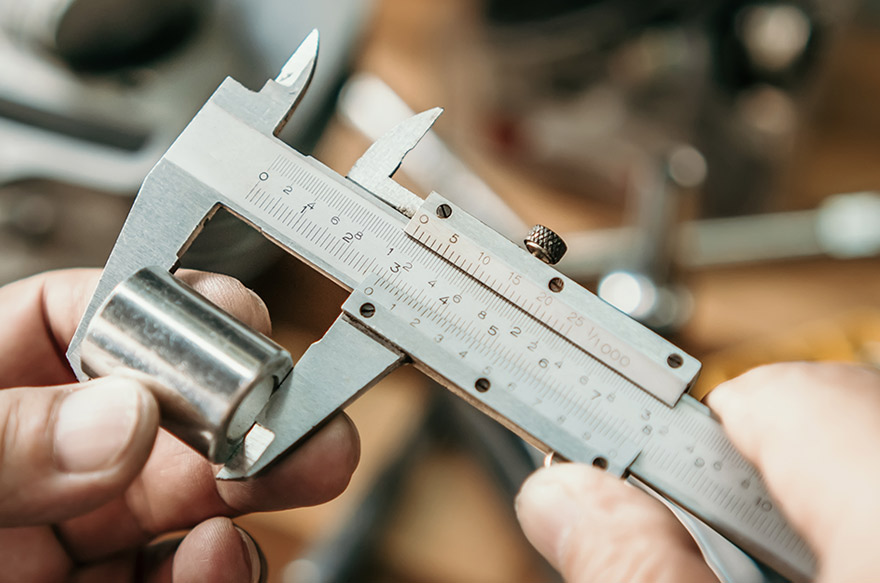
- Automated manufacturing processes must carefully manage measurement uncertainty to ensure consistent product quality.
- Advanced techniques, such as Monte Carlo simulation, sensitivity analysis, and machine learning, are used to reduce and manage measurement uncertainty in complex automated systems.
- Recent innovations, including predictive models and new instruments, have significantly improved the accuracy and repeatability of measurements, reducing overall uncertainty.
Automation in manufacturing relies heavily on precise and accurate data, making the management of measurement uncertainty crucial. As processes become more automated and involve less human intervention, metrologists must carefully monitor uncertainties throughout the production cycle. Even small errors can accumulate over time, leading to significant issues in the final product. Ensuring traceability and appropriately propagating uncertainty are essential steps in maintaining product quality.
Challenges in managing measurement uncertainty include finding reference artifacts with sufficiently low uncertainty and accounting for environmental factors like temperature fluctuations. Changes in suppliers’ reported uncertainties can also impact the overall uncertainty of measurements. Recognizing and minimizing these uncertainties are key responsibilities of metrologists, who must also differentiate between precision and accuracy in their measurements. While precision refers to the consistency of repeated measurements, accuracy is about hitting the true value.
Recent advancements have introduced tools and techniques that help reduce measurement uncertainty. Predictive models, digital adjustable force systems, and laser measurement technologies are among the innovations that enhance measurement accuracy and repeatability. Advanced methods such as Monte Carlo simulation, Bayesian inference, and machine learning techniques are also applied to manage uncertainty in complex automated systems. These methods help operators identify the most significant sources of uncertainty and optimize processes for better accuracy and reliability.
Leave a Reply
You must be logged in to post a comment.