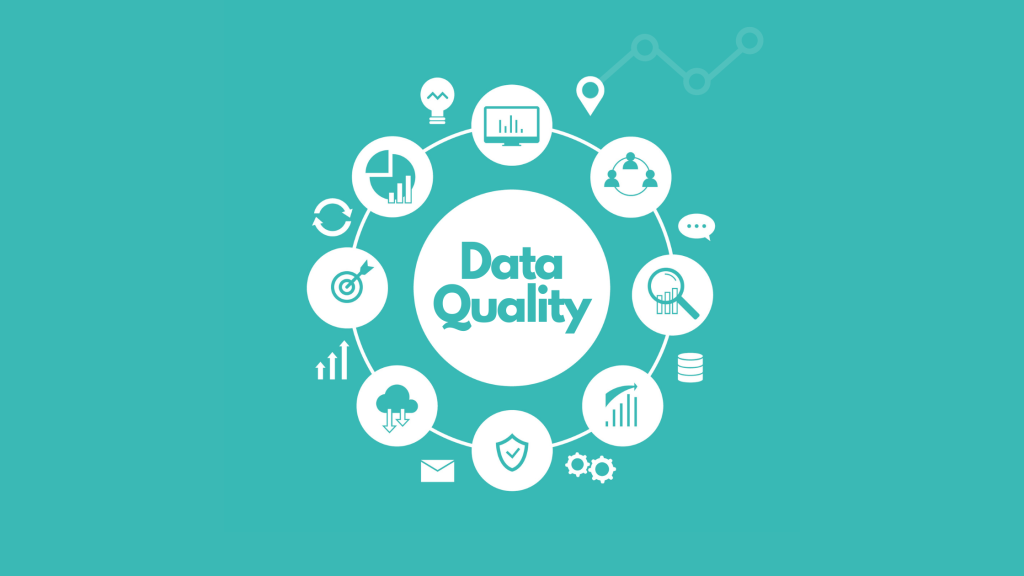
- Using machine learning models trained on clean data, this approach enhances data cleaning by leveraging unstructured textual inputs such as titles or descriptions to predict and correct errors in structured data.
- The proposed method integrates Natural Language Processing (NLP) techniques with machine learning classifiers and applies constraint-based filtering to improve training data quality and model performance.
- Experiments with real-world datasets show that the hybrid strategy, combining the ML-based model with traditional rule-based engines like Parker, yields strong results, with precision up to 0.88 and recall up to 0.94.
This paper introduces a machine learning-based strategy to improve data cleaning by integrating unstructured textual data with traditional structured datasets. Recognizing that structured data often contains errors due to inconsistencies, omissions, or conflicts, the authors propose leveraging textual descriptions, such as product titles or clinical trial summaries, to predict and correct inaccurate entries. The process begins by identifying “clean” data that adheres to integrity constraints like functional dependencies and selection rules. These clean samples are used to train machine learning models that learn mappings between unstructured text and structured attribute values.
The ML models rely on various NLP preprocessing techniques, such as Bag-of-Words (BOW), TF-IDF, and BERT embeddings, to transform unstructured text into usable features. These features then feed into base learners like XGBoost, Logistic Regression, Naïve Bayes, or Artificial Neural Networks to predict structured attribute values. During the data repair phase, the models output probability distributions for each potential value. A configurable confidence threshold determines whether a predicted value is used to replace a potentially incorrect one, ensuring that replacements are made only when the model has high confidence.
The paper also explores a hybrid approach that combines the ML model with a rule-based system called the Parker engine. This combined method either uses the ML predictions as inputs to Parker or uses Parker-cleaned data as training input for the ML model. The hybrid strategy generally improves performance, showing how integrating rule-based and learning-based systems can yield better data repair outcomes. Experimental evaluations using datasets related to clinical trials and allergen labeling validate the method’s effectiveness. Among several tested models, the combination of TF-IDF with XGBoost performs best overall in both prediction accuracy and repair robustness.
Performance evaluations demonstrate that using constraint-verified training data improves precision and recall metrics. In particular, the best models achieve a precision of 0.88 and a recall of 0.94 in some cases. While training with BERT embeddings underperformed due to the factual rather than narrative nature of the text, simpler embeddings like TF-IDF, when paired with XGBoost, yielded more reliable results. The paper concludes by emphasizing the value of using textual data to inform structured data cleaning and the strength of combining AI techniques with constraint-based frameworks for scalable and accurate data repair.
Leave a Reply
You must be logged in to post a comment.