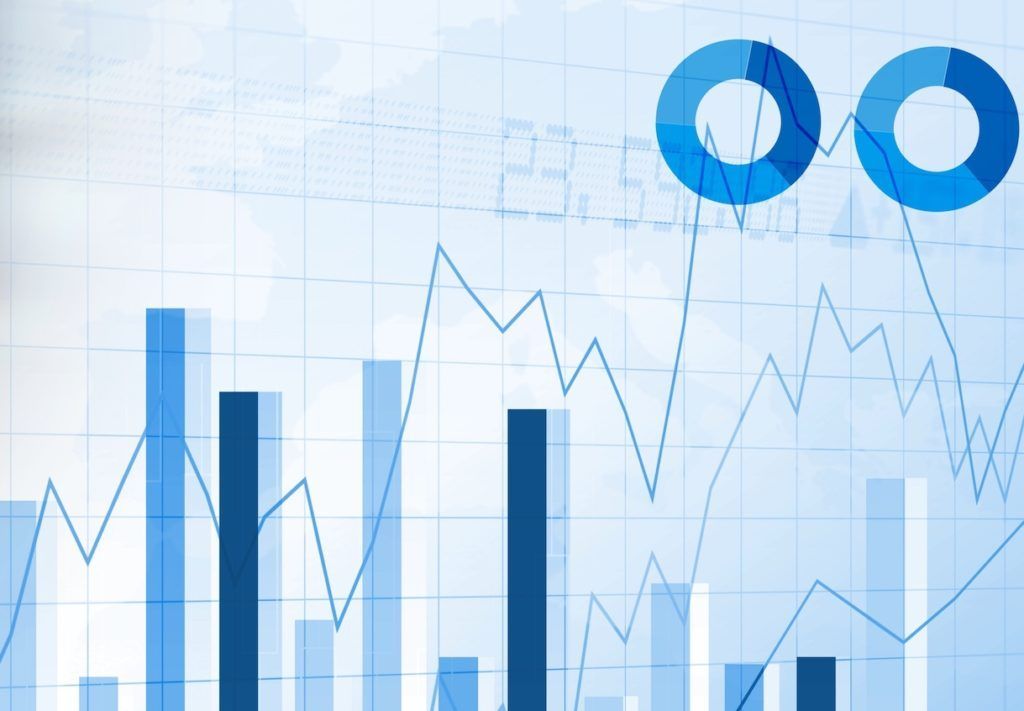
- Statistical confidence represents the probability that a statistical method correctly infers population parameters.
- Confidence intervals account for sampling variation and other uncertainties in estimates.
- Statistical confidence is a long-run probability based on repeated sampling under identical conditions.
Statistical confidence refers to the probability that a statistical method correctly infers something about a population based on a sample. This concept is often misunderstood. Confidence is not a guarantee but a long-run probability that the technique will capture the proper population parameter if repeated many times. Typical confidence levels, such as 95%, imply a 5% risk of drawing an incorrect conclusion.
For example, estimating the proportion of orange M&Ms in a bag involves sampling and calculating a confidence interval. If 21 experimenters each estimate this proportion, their results will vary due to sampling variation and other uncertainties. A 95% confidence interval means that 95% of such intervals, calculated from repeated samples, will contain the proper proportion.
An experiment involving 21 independent bags of M&Ms illustrated this concept. Each experimenter calculated the proportion of orange M&Ms and the corresponding 95% confidence interval. One interval, calculated by an experimenter with bag 1, correctly captured the proper proportion, around 20%. However, another interval underestimated it, demonstrating the 5% risk associated with 95% confidence.
In summary, statistical confidence is a long-run measure of a method’s reliability in capturing the proper population parameter. It reflects the probability that repeated sampling under identical conditions will yield correct conclusions about the population or process.
Leave a Reply
You must be logged in to post a comment.