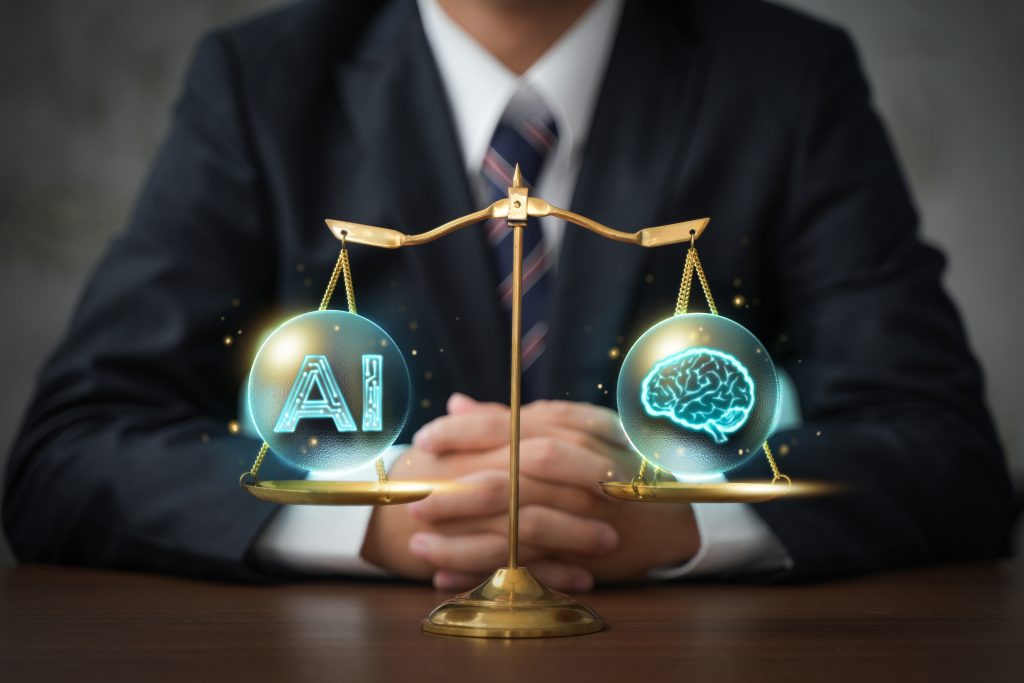
- There is a complex relationship between artificial intelligence (AI), specifically generative AI, and data quality management, with a crucial role of high-quality data in developing successful AI models.
- The discourse around AI’s capability to improve data quality presents a conundrum: while enhancing data quality can lead to better AI models, those improved models, in turn, have the potential to elevate data quality.
- AI and data quality improvement are experiencing a symbiotic relationship, each driving the importance of the other.
The full article delves into the complex relationship between artificial intelligence (AI), specifically generative AI, and data quality management. What is the crucial role of high-quality data in developing successful AI models? We can draw parallels to how healthy food benefits humans, suggesting that data acts as sustenance for AI. Despite the excitement surrounding AI’s potential to enhance various information processes, data quality emerges as a pivotal factor in maximizing AI’s effectiveness. The article references several sources that underline the importance of data quality, including research that identifies new data quality dimensions necessitated by AI, such as model bias, which poses challenges not previously considered by business and IT managers.
The discourse around AI’s capability to improve data quality presents a conundrum: while enhancing data quality can lead to better AI models, those improved models, in turn, have the potential to elevate data quality. This creates a positive feedback loop, raising questions about the eventual convergence of AI and data quality. Talburt examines practical ways AI might indirectly benefit data quality by facilitating traditional data quality processes like profiling and validation through generative models. However, he also points out AI’s direct contributions, particularly in managing unstructured data, constituting a significant portion of organizational data. AI’s ability to perform named entity recognition (NER) more accurately and generate realistic synthetic data for system testing are highlighted areas where AI directly contributes to data quality improvement.
AI and data quality improvement are experiencing a symbiotic relationship, each driving the importance of the other. While AI elevates data quality management’s significance within organizations, it also benefits from rigorous data quality improvement. The intersection of AI and data quality management is evolving, with standards like ISO 8000 Part 61 providing a framework for integrating data quality management into data governance programs. This analysis underscores the intertwined futures of AI and data quality, suggesting that advancements in one area will likely catalyze progress in the other, ultimately benefiting the broader field of data management and governance.
Leave a Reply
You must be logged in to post a comment.