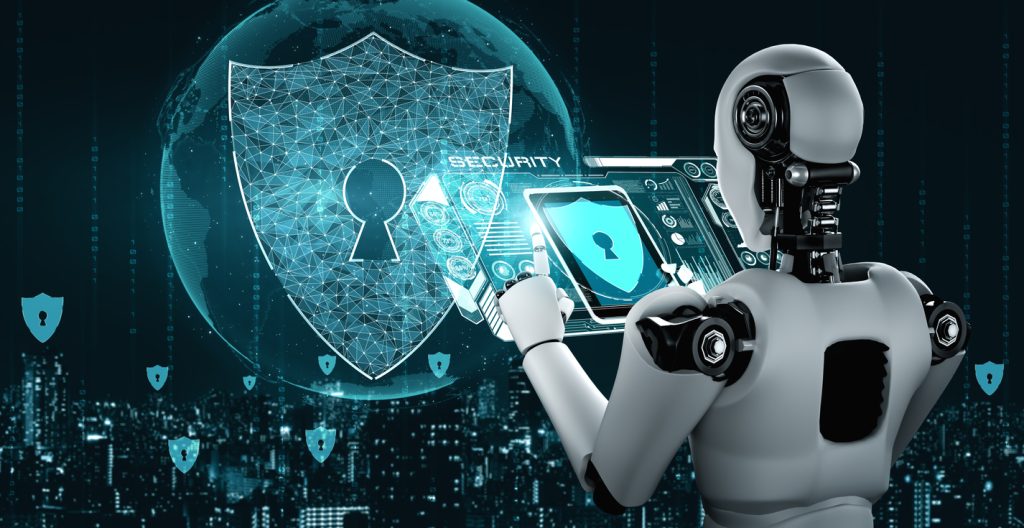
- AI-driven quality control significantly improves defect detection, reducing reliance on human inspection and increasing accuracy to near 99%.
- Manufacturers adopting AI-based inspections can lower defect escape rates, reduce operational costs, and improve overall product quality with faster deployment and adaptability.
- Demonstrating AI’s value through proof-of-value pilots helps companies justify investment and gain stakeholder buy-in for long-term integration.
AI transforms quality control in electronics manufacturing by improving accuracy, reducing costs, and increasing efficiency. Traditional quality control methods rely heavily on human inspectors, who typically catch about 80% of defects, whereas AI-powered inspection systems can achieve up to 99% accuracy. AI’s ability to process large datasets, adapt to new product designs, and detect both known and novel defects makes it a powerful tool for manufacturers. Unlike traditional computer vision systems, which require extensive programming and are limited to pre-defined failure modes, AI-based systems learn from data and continuously improve, making them more flexible and scalable.
A key advantage of AI in quality control is its ability to reduce “dark yield”—defects that escape factory inspections and cause failures in the field. A case study involving a $50 billion communications company demonstrated how AI inspection systems helped detect critical defects in first-responder radios that had previously gone unnoticed. The company identified missing labels and switched buttons by running a proof-of-value pilot with 1,000 units, proving AI’s effectiveness in preventing costly quality escapes. This resulted in measurable savings, as AI reduced inspection times and eliminated the need for additional operators, achieving a return on investment within one month.
Despite AI’s clear benefits, manufacturers face adoption challenges, including resistance to change and concerns about integration with existing workflows. To address these barriers, companies should focus on demonstrating AI’s tangible impact through proof-of-value pilots, which quantify improvements in defect detection, cost savings, and efficiency gains. Quality leaders are uniquely positioned to drive this transformation, ensuring AI is not just an experimental technology but a strategic asset for improving manufacturing precision. The question is no longer whether AI should be adopted but how quickly manufacturers can implement it to gain a competitive advantage.
Leave a Reply
You must be logged in to post a comment.