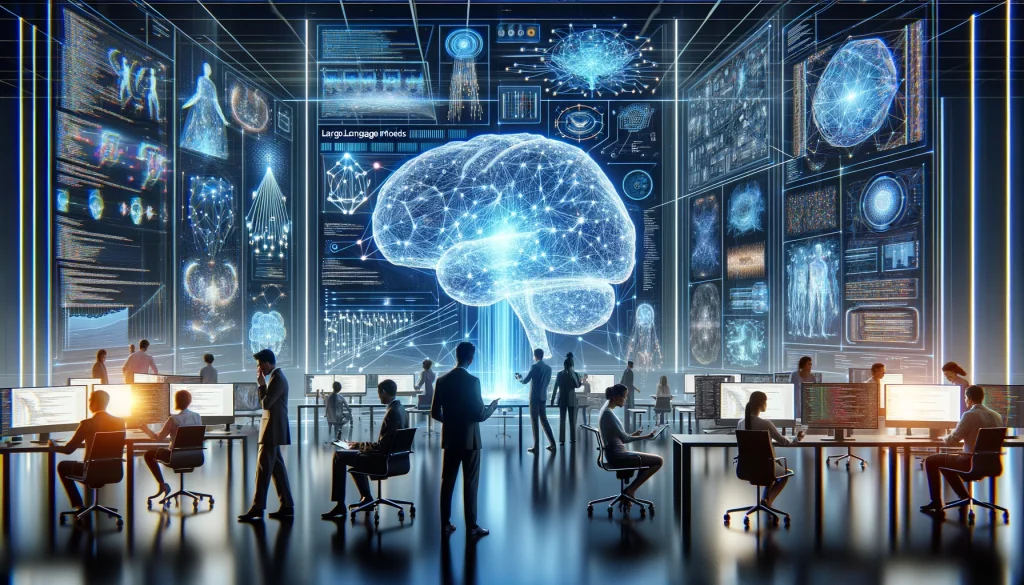
- The rapid adoption of large language models (LLMs) in enterprise environments in 2024 has brought new cybersecurity challenges to the forefront, particularly concerning data leakage and the complexities introduced by integrating LLMs with cloud services.
- Addressing the security concerns associated with LLMs requires a multifaceted approach that includes robust access controls, user authentication, encryption, data loss prevention, and network security.
- The integration of LLMs into cloud services without sufficient security validations poses direct attack vectors, as Itamar Golan of Prompt Security notes.
The rapid adoption of large language models (LLMs) in enterprise environments in 2024 has brought new cybersecurity challenges to the forefront, particularly concerning data leakage and the complexities introduced by integrating LLMs with cloud services. With enterprises increasingly hosting multiple iterations of LLMs across their cloud environments, the risk landscape expands, making it difficult for CISOs to protect their organizations fully. Despite the location of LLM hosting—be it cloud-based, on-device, or on-premises—the exposure to cloud-related threats remains significant. Furthermore, the proliferation of shadow LLMs, where employees access public models like ChatGPT and BingChat/Co-Pilot for various tasks, exacerbates the risk of sensitive corporate data leakage through these public platforms.
Addressing the security concerns associated with LLMs requires a multifaceted approach that includes robust access controls, user authentication, encryption, data loss prevention, and network security. Mitigating unauthorized LLM use is complex, especially when proprietary or confidential data is inadvertently fed into these models. George Chedzhemov from BigID emphasizes the importance of data discovery as a foundational step in any data risk remediation strategy, highlighting the challenges in protecting data that is either lost, over-permission, or unknown to the organization. Similarly, Brian Levine from Ernst & Young points to the difficulty in controlling shadow LLMs, especially when employees use their devices, making it harder to differentiate between AI-generated and user-generated content.
The integration of LLMs into cloud services without sufficient security validations poses direct attack vectors, as Itamar Golan of Prompt Security notes. This rush to integrate LLMs for rapid feature deployment can bypass essential security checks, leaving cloud environments vulnerable to attacks. Attackers are expected to target LLM systems, exploiting unsecured infrastructure for their own purposes, including data mining and advanced phishing campaigns, as Bob Rudis from GreyNoise Intelligence explained. The article suggests that security teams must now incorporate AI awareness into all security decisions, focusing on AI-specific vulnerabilities and ensuring data security and compliance. This new security paradigm underscores the need for closer collaboration between AI developers and security teams, as well as continuous re-evaluation of AI models to address potential risks, biases, and vulnerabilities inherent in using LLMs and their integration into cloud infrastructures.
Leave a Reply
You must be logged in to post a comment.